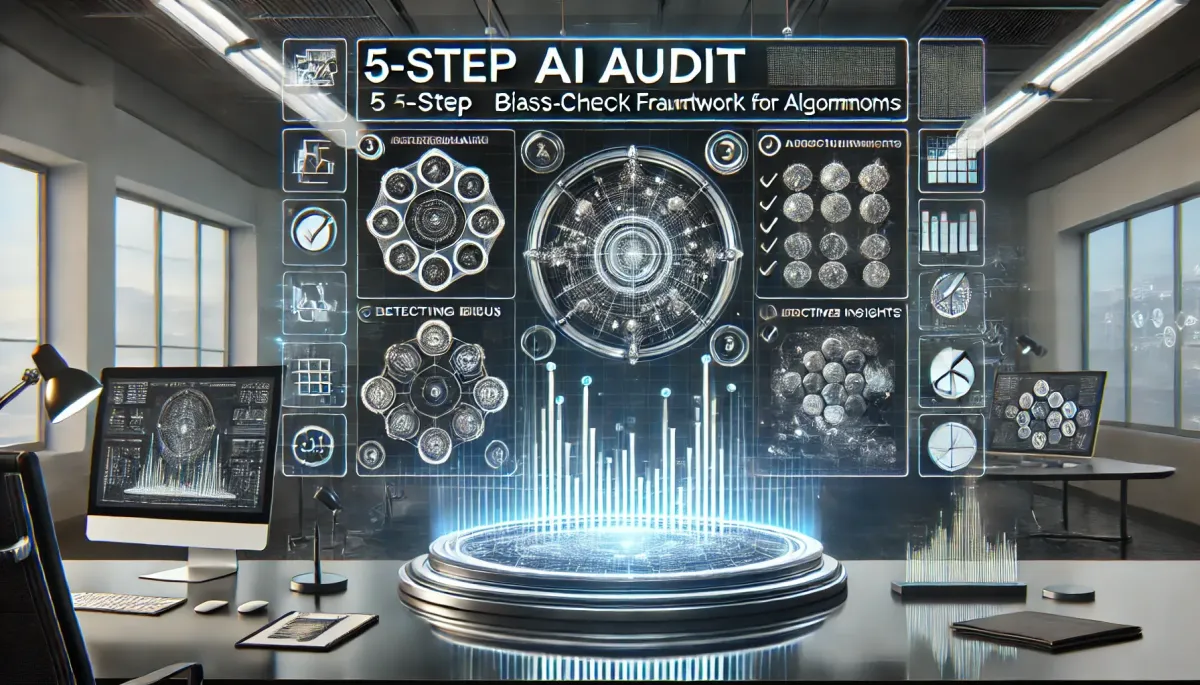
Audit Your Algorithm – A 5-Step Bias-Check Framework
Audit Your Algorithm – A 5-Step Bias-Check Framework
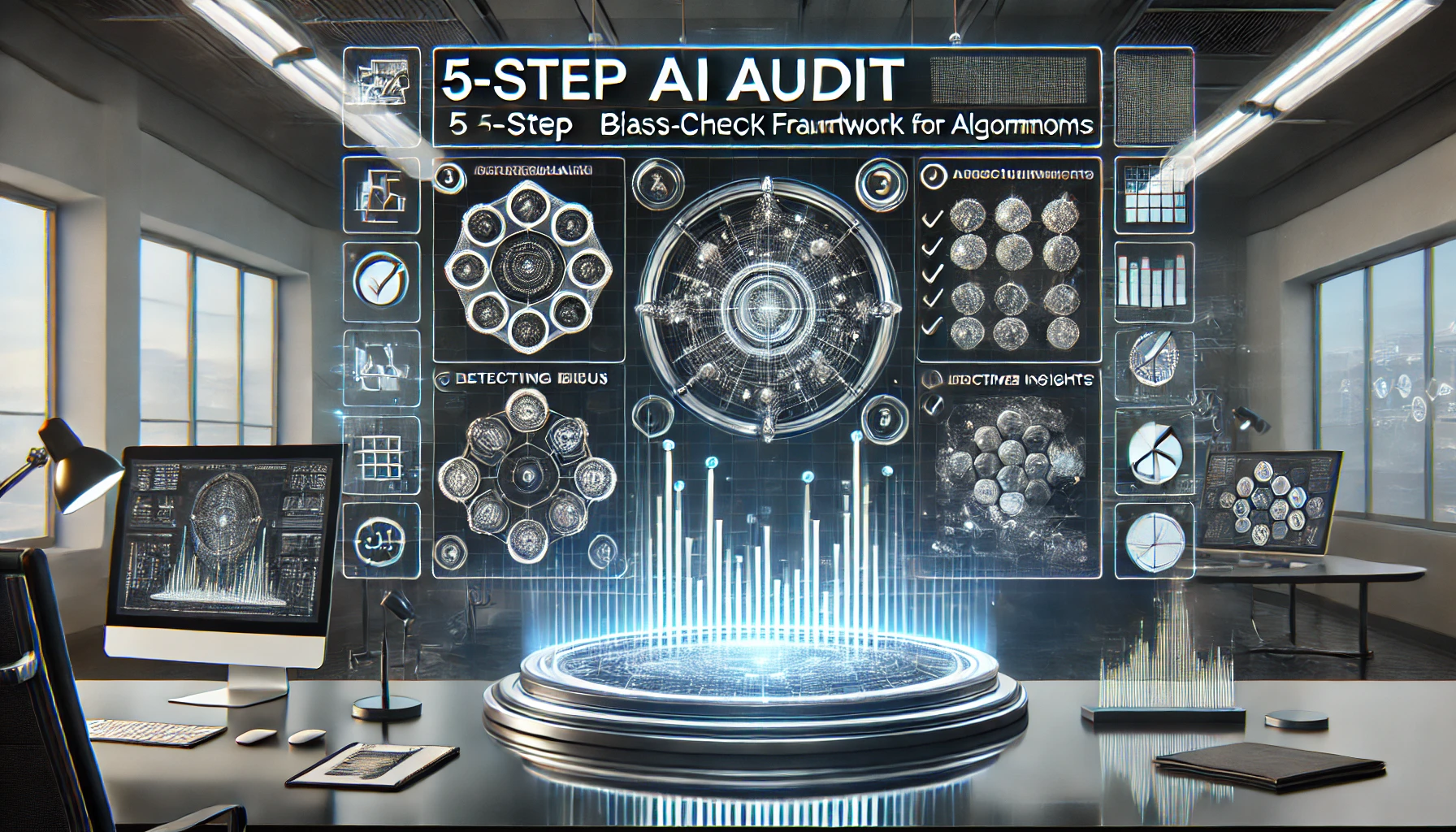
Introduction
AI is transforming recruitment, but here’s the catch—it can also amplify bias if left unchecked. A biased AI means biased hiring decisions, leading to skewed talent pools, compliance risks, and reputational damage.
So, how do you ensure your AI hiring tools are fair, ethical, and bias-free? Enter the 5-Step Bias-Check Framework—a structured approach to auditing recruitment AI for fairness and compliance.
Why AI Bias is a Major Hiring Concern
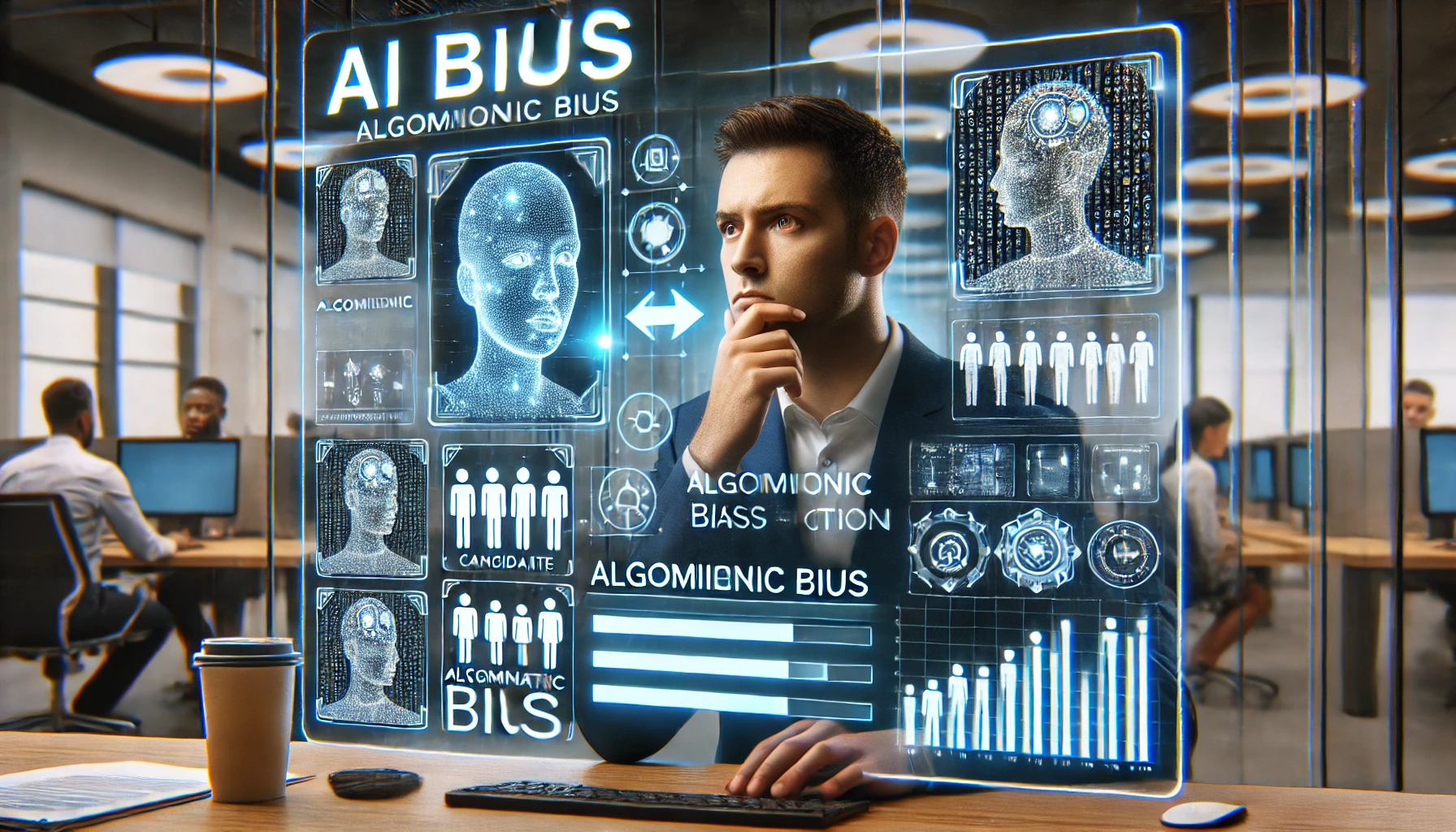
Before we dive into solutions, let’s break down the risks of unchecked AI bias in recruitment:
❌ Discriminatory Hiring Patterns – AI trained on biased historical data replicates past hiring mistakes.
❌ Legal & Compliance Issues – Non-compliance with DEI regulations can lead to lawsuits and fines.
❌ Diversity Blind Spots – AI may unintentionally screen out diverse candidates based on irrelevant factors.
❌ Damage to Employer Brand – A biased hiring process can lead to bad press and loss of candidate trust.
Case Study: "A major financial firm discovered that its AI hiring tool favored male candidates due to historical hiring patterns, leading to a complete system overhaul."
The 5-Step Bias-Check Framework
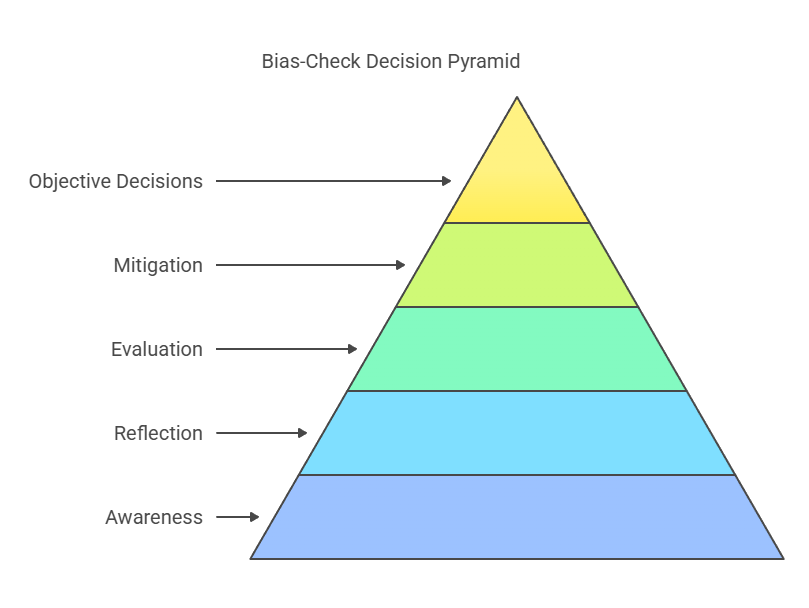
To ensure fair and ethical hiring decisions, follow this bias-audit process:
1. Data Integrity & Source Review
📊 Audit AI training data for potential biases—where does it come from? Are past hiring decisions shaping future ones unfairly?
📊 Remove historical hiring biases by diversifying datasets.
📊 Normalize data so that demographic factors don’t skew AI recommendations.
2. Algorithm Transparency & Explainability
🔍 Understand how AI makes hiring decisions—no more ‘black box’ algorithms!
🔍 Enable AI interpretability tools to show why certain candidates are prioritized.
🔍 Ensure compliance with EEOC, GDPR, and other fair hiring laws.
3. Bias Detection & Testing
🛠️ Run bias-detection tools to analyze AI screening results.
🛠️ Test AI recommendations using synthetic candidate profiles from diverse backgrounds.
🛠️ Compare human vs. AI hiring decisions to detect discrepancies.
4. Continuous Monitoring & Adjustment
📈 Regularly audit AI to identify shifts in bias over time.
📈 Adjust weightings & filters to reduce unintended discrimination.
📈 Gather candidate feedback to ensure AI-driven decisions are fair and explainable.
5. Human-AI Collaboration
👥 Keep humans in the loop—AI should assist, not replace, recruiter judgment.
👥 Train hiring teams on AI oversight and ethical recruitment practices.
👥 Use AI recommendations as insights, not absolute decisions.
Example: "An AI-powered resume screener was filtering out candidates with career gaps, disproportionately affecting women returning from maternity leave. The company adjusted its algorithm to weigh skills over gaps, improving diversity by 20%."
Best Tools for AI Bias Auditing
Want to audit your hiring AI? Here are top AI fairness tools:
🔹 IBM AI Fairness 360 – Open-source bias detection for AI models. 🔹 Pymetrics Audit AI – Bias-checking for AI hiring decisions. 🔹 Textio Fairness Metrics – Detects bias in job descriptions & hiring copy. 🔹 HiredScore Fair Hire Index – AI-driven fair hiring compliance tracker.
Fun Fact: "Organizations that regularly audit AI for bias see a 30% increase in diversity hiring outcomes."
Common Pitfalls & How to Avoid Them
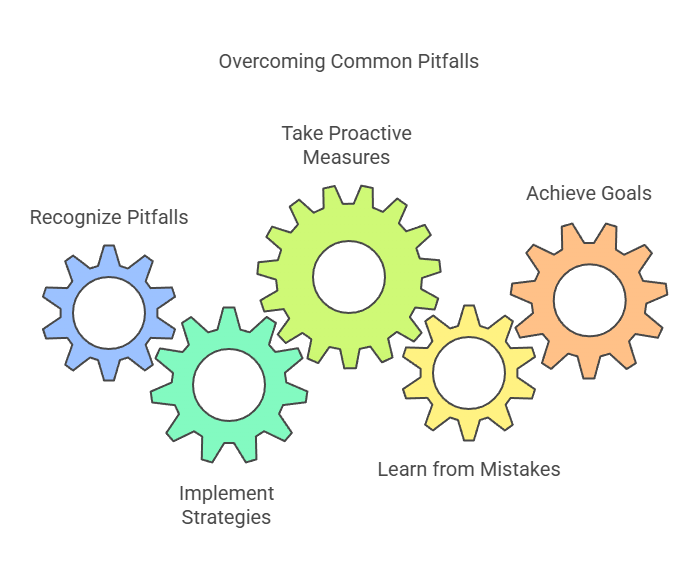
Even with audits, AI hiring can still go wrong. Here’s what NOT to do:
❌ Assuming AI is Neutral – AI inherits biases from data—it’s not naturally fair.
❌ Skipping Regular Audits – AI bias evolves over time—what’s fair today may not be fair tomorrow.
❌ Relying 100% on AI – Always have human oversight in hiring decisions.
❌ Ignoring Candidate Feedback – Transparency builds trust—explain how AI decisions are made.
Case Study: "A tech company noticed its AI preferred Ivy League graduates—after an audit, they broadened their hiring criteria, improving social mobility hires by 25%."
Final Thoughts: Ethical AI = Smarter Hiring
Bias in AI isn’t a one-time fixo—it’s an ongoing process that requires active oversight, audits, and adjustments. The best hiring teams don’t just use AI—they shape it into a tool for fair and diverse hiring.
By implementing bias audits, transparency protocols, and human-AI collaboration, recruiters can make hiring decisions that are not only efficient but also ethically sound.
🚀 Next Up: When AI Discriminates – Fixing a Broken Recommendation Engine 👀
How are you ensuring fair AI hiring decisions in your company? Let’s discuss in the comments!